Years prior to ChatGPT’s existence, Nick Vincent delved into the world of AI’s reliance on human-generated data. He noted that while researchers and tech companies emphasized their advanced algorithms, they often overlooked the significance of the underlying data. This perspective is gradually shifting as the value and sources of data for AI models become a prominent concern.
Prominent AI models like GPT-4, PaLM 2, and Llama 2 have been constructed using vast amounts of online content, raising questions about copyright violations and fair compensation for data contributors. Evaluating the worth of individual data pieces in a sea of information has become a challenge. This quandary was spotlighted in a recent AI blog by Benedict Evans, underscoring that these models demand access to a comprehensive spectrum of data, rendering each individual contribution less distinct.
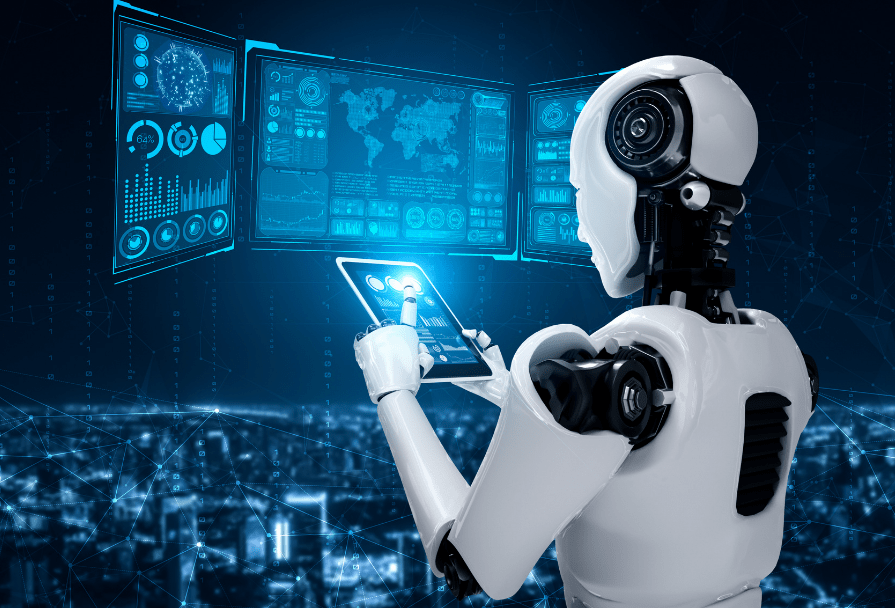
Vincent coined the term “Data Leverage” to describe the concept that communities should comprehend the importance of their data contributions for AI models and negotiate payment accordingly. This month, two significant papers entered the fray, aiming to address different aspects of this issue.
“If we know that all our books together are responsible for half the ‘goodness’ of ChatGPT, then we can put a value on that,” he said. “That was a fringe concept a few years ago and it is becoming more mainstream now. I’ve been beating this drum for years, and it’s finally happening. I’m shocked to see it.”
Anthropic, an advanced AI company, unveiled a paper outlining an innovative method for efficiently swapping data and gauging model performance changes. This addresses a previously expensive challenge in modifying large language models.
“When an LLM outputs information it knows to be false, correctly solves math or programming problems, or begs the user not to shut it down, is it simply regurgitating (or splicing together) passages from the training set? Or is it combining its stored knowledge in creative ways and building on a detailed world model?” the Anthropic researchers wrote. “We believe this work is the first step towards a top-down approach to understanding what makes LLMs tick.”
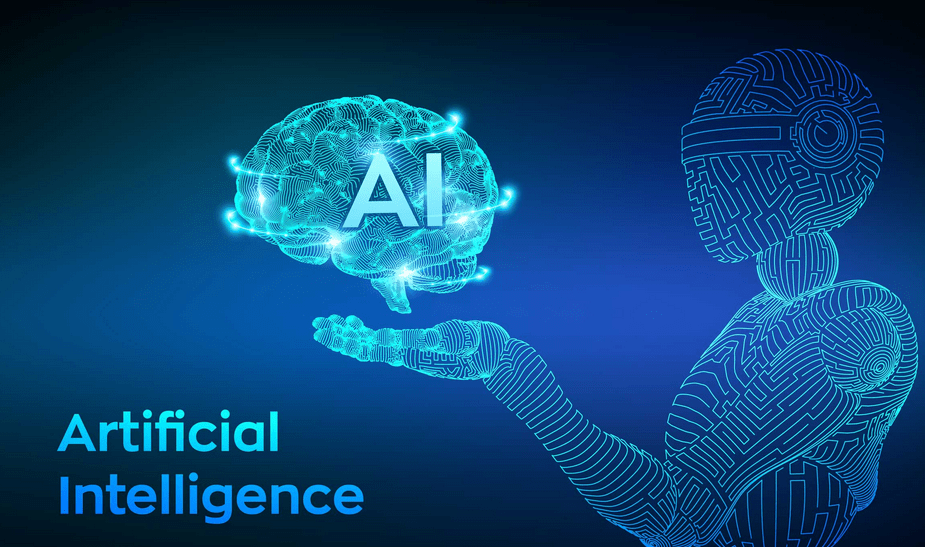
Another effort, by SILO (a new language model that was developed by researchers at the University of Washington in Seattle) was led by researchers from the University of Washington, UC Berkeley, and the Allen Institute for AI, introduced a new language model. SILO not only focuses on reducing legal risks by allowing data removal but also offers a mechanism to measure the impact of specific data on an AI model’s output. This approach provides an avenue for data owners to receive proper credit or direct payment when their data contributes to predictions.
The researchers examined the influence of specific data using the example of J.K. Rowling’s Harry Potter books. They demonstrated that while AI models rely heavily on high-quality human-generated content under copyright, performance degrades when trained solely on low-risk, out-of-copyright material.
Through meticulous experimentation with different datasets that excluded portions of the Harry Potter series, the researchers showed that AI models’ performance suffered without those specific content pieces. This “leave-out” analysis highlighted that AI models struggle to answer questions accurately when trained content is missing.
The SILO study doesn’t aim to profit from copyrighted material but rather demonstrates the feasibility of building powerful AI models while mitigating legal risks. The model was trained on low-risk public domain text, and high-risk data, including copyrighted books, was introduced during the inference stage. This innovative approach carries legal benefits, allowing authors to opt out and providing a means to attribute sentences to authors in the results.
Overall, the research underscores the complex interplay between AI, data, copyright, and compensation, as the field strives to strike a balance between technological advancement and ethical considerations.