Vehicle pollution poses a substantial threat to both global and local air quality, emitting harmful pollutants like carbon monoxide, nitrogen oxides, particulate matter, and hydrocarbons. In the US, transportation-related greenhouse gas emissions have surged, contributing 29% of total emissions, surpassing other sectors.
To address this, Yu Yang, a computer science and engineering assistant professor at Lehigh University, leads a team developing a traffic light management system using machine learning. Their approach aims to foster smoother traffic flow, minimizing vehicle emissions. Stoplights in congested areas significantly exacerbate localized air pollution, especially affecting individuals with respiratory conditions like asthma.
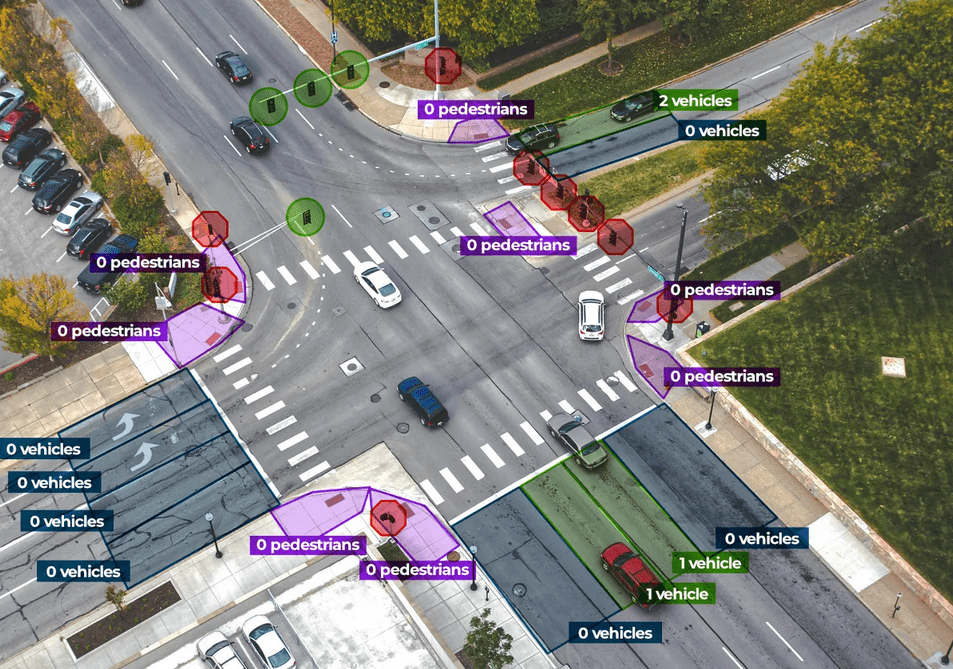
The researchers adopt a three-pronged strategy. First, they create affordable, mobile air-quality sensors to identify high-pollution zones, considering the environmental needs of diverse locations. Then, they develop a spatial-temporal graph diffusion learning model to analyze traffic and air quality variations across different areas and times, focusing on Newark, New Jersey.
“We’ll use those data to then develop a spatial-temporal graph diffusion learning model to determine the traffic situation in our test-bed city of Newark, New Jersey,” said Yang. “In other words, what is both the traffic and the air pollution like at different points of time in different locations?”
Utilizing reinforcement learning, the team explores how adjusting traffic signals can improve air quality, integrating a social component into traffic control—a novel approach. This study emphasizes both technical and social angles to tackle real-world issues.
“This is the first project of its kind to incorporate a social component into a traffic control system,” said Yang. “We’re taking both a technical and a social perspective to solve a real-world problem.”
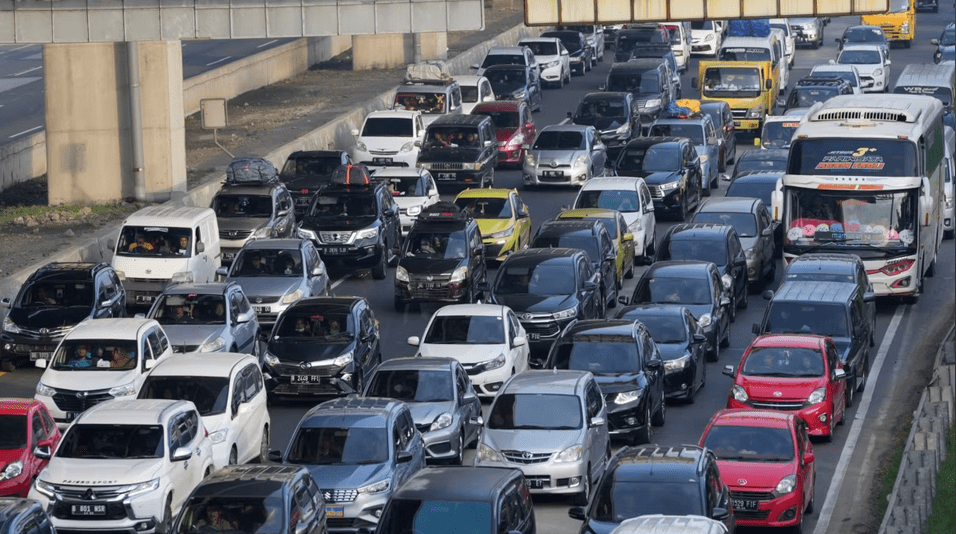
Moreover, the project considers human behavior in transportation choices within urban settings, challenging assumptions that people select transportation randomly. Yang’s team believes incorporating this data will enhance the algorithm’s accuracy, providing insights into actual user-system interactions.
The ultimate goal is to build a web-based system showing location-specific air quality, empowering city dwellers to make informed decisions about their activities based on environmental conditions. Complementing this, a dynamic traffic management model allows city officials to adapt signal functioning in real-time, mitigating air pollution in the most affected areas.
This innovative initiative not only focuses on improving traffic systems but also integrates a social understanding of urban transportation, aiming to empower individuals with knowledge to make environmentally conscious choices while providing officials with tools to actively mitigate pollution. Yang’s multifaceted approach seeks to bridge the gap between technological advancements and societal needs in combating vehicle pollution.