The tech industry now relies heavily on artificial intelligence (AI), which has led to businesses including AI-powered features in their products. But the increased use of AI has also sparked questions about how energy-efficient the supporting infrastructure is. In response to this problem, engineers at Northwestern University in the United States have created a novel transistor design that not only enables shrinking but also increases the energy efficiency of AI operations by 100 times.
Although silicon-based chips have historically served as the basis for AI technology, it has become clear that these chips have drawbacks, particularly with regard to efficiency and energy consumption. Energy-intensive steps in the data processing cycle include gathering data, sending it to the cloud for analysis, and finally returning the results to the user. The Northwestern University group, under the direction of Professor Mark Hersam, investigated substitute materials for transistors in order to combat this.
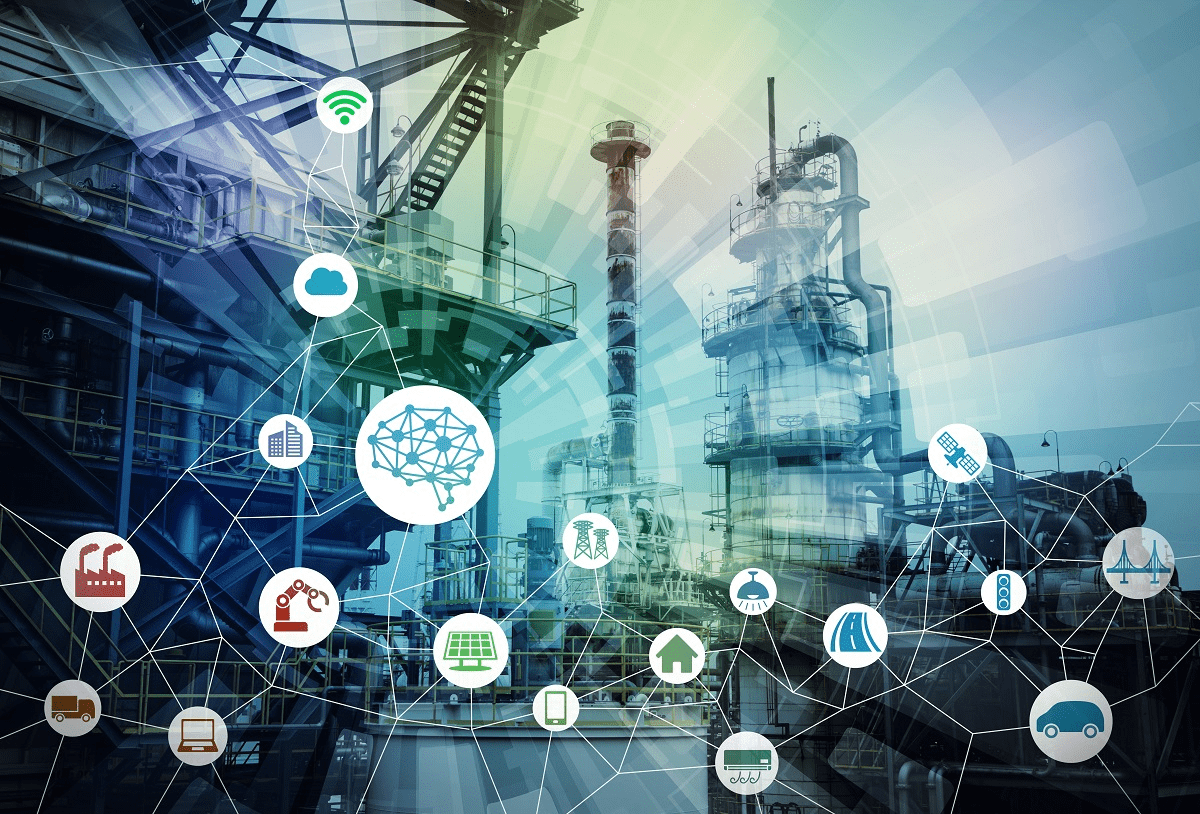
They opted for a design utilizing two-dimensional molybdenum disulfide and one-dimensional carbon nanotubes, allowing the transistors to be reconfigured for different analysis steps. This design drastically reduced the number of transistors needed and the energy consumed while enabling miniaturization. Impressively, the analysis could be integrated into regular wearable devices.
The increased security and privacy of personal data is a major benefit of this strategy. Wearable technology is an example of how local data processing limits data transfer and so lowers the danger of data breaches. By teaching AI to analyze electrocardiogram (ECG) data, the researchers effectively showcased the potential of their gadget. They achieved remarkable accuracy with just two transistors, a feat that would normally need at least 100 silicon transistors.
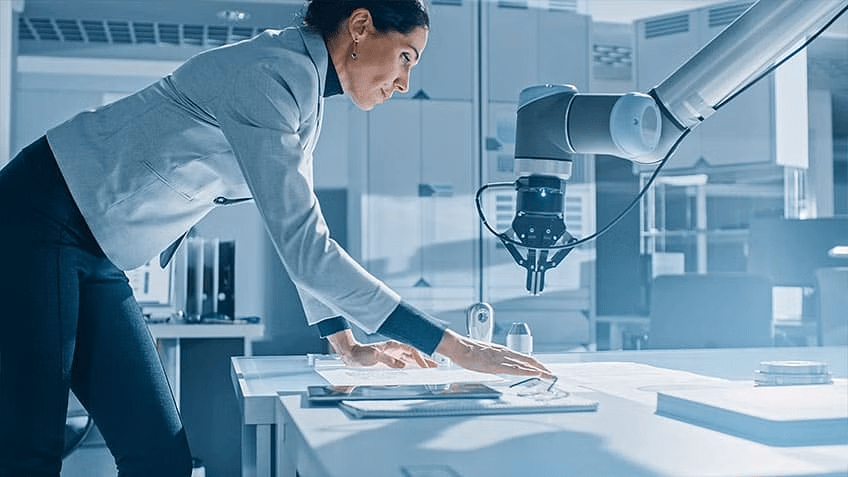
The future holds promising possibilities, envisioning the integration of these efficient devices into everyday wearables, enabling real-time applications without burdening the power grid. This innovation stands as a remarkable step toward sustainable and efficient AI technology, revolutionizing the landscape of AI-powered devices and applications.