Fingerprint analysis has long been a cornerstone in forensic and security applications due to the distinctive and enduring nature of these patterns. The claim that no two individuals, not even identical twins, share the same fingerprint pattern underscores its significance. However, recent research from Columbia University suggests that artificial intelligence (AI) might challenge conventional beliefs in forensic fingerprint identification.
The crux of the matter lies in the difficulty of matching intra-person fingerprints, those from different fingers of the same person. The research team asserts that, contrary to the established belief in fingerprint uniqueness, the fingerprints of the same person are not truly unique, and the traditional comparison methods might be flawed.
The current fingerprint analysis involves examining friction ridge patterns, categorized as loops, whorls, and arches. Analysts rely on minutiae, specific ridge characteristics, for identification and comparison. The AI model developed by Columbia University, however, adopts a different approach. It assesses binary patterns, ridge orientation, ridge density, and minutiae, with a focus on the singularity – the swirls and loops near the fingerprint center.
The researchers argue that minutiae analysis, although accurate for same-finger matching, is less reliable for cross-finger matching within the same person. According to their findings, ridge orientation was a more robust indicator of intra-person fingerprint similarities than minutiae maps.
The AI model was trained using a public US government database containing around 60,000 fingerprints and 525,000 images. The researchers reported a 99.99 percent confidence in the model’s ability to match intra-person fingerprints. Importantly, the model demonstrated consistent performance across different genders and racial groups, performing best when trained with samples from all groups.
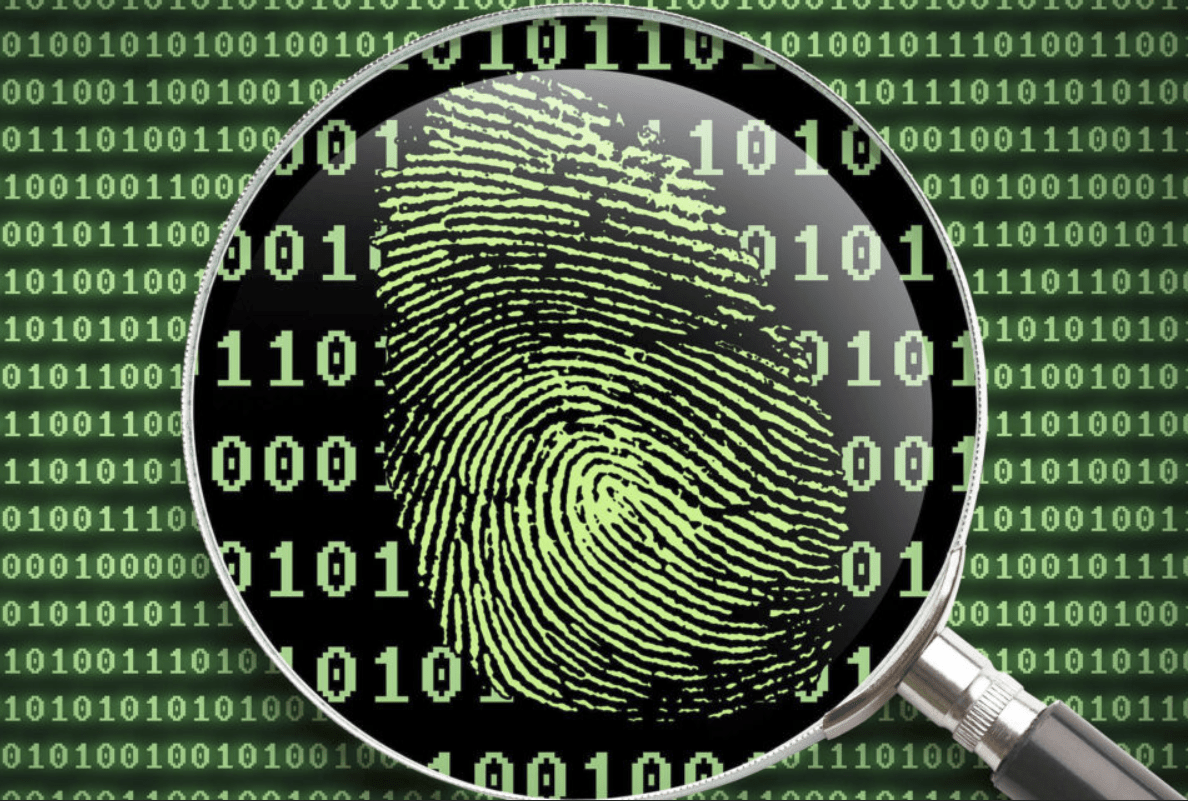
The potential applications of this AI-led fingerprint analysis are extensive. The researchers envision its use in prioritizing leads, exonerating innocent suspects, and generating new leads for cold cases. They express optimism about the model’s future performance with more extensive training datasets.
However, the research has faced criticism, particularly from the established forensics community. A well-established forensics journal initially rejected the paper, citing the widely held belief that every fingerprint is unique. The debate hinges on whether AI can produce results reliable enough to serve as conclusive evidence in criminal convictions.
In conclusion, the Columbia University research challenges the conventional understanding of fingerprint uniqueness by leveraging AI to analyze intra-person fingerprints. While the model shows promise in achieving high accuracy, it faces skepticism from the forensics community, highlighting the ongoing debate regarding the role of AI in reshaping forensic practices.