Text-to-image artificial intelligence (AI) systems have revolutionized visual content creation but raised significant legal concerns, particularly regarding copyright infringement. Lawsuits have been filed against models such as Imagen by Google, as well as Stability AI and Midjourney, accusing them of utilizing copyrighted images without authorization for training purposes. This presents a legal challenge for both companies like Alphabet and artists who discover their work reproduced without consent.
In response to this dilemma, researchers from the University of Texas at Austin and the University of California, Berkeley, have devised an innovative remedy. They’ve developed an original solution called Ambient Diffusion, a generative AI framework based on diffusion, trained exclusively on heavily corrupted images. This novel approach decreases the likelihood of the model memorizing and duplicating original works, thereby circumventing potential copyright infringement issues.
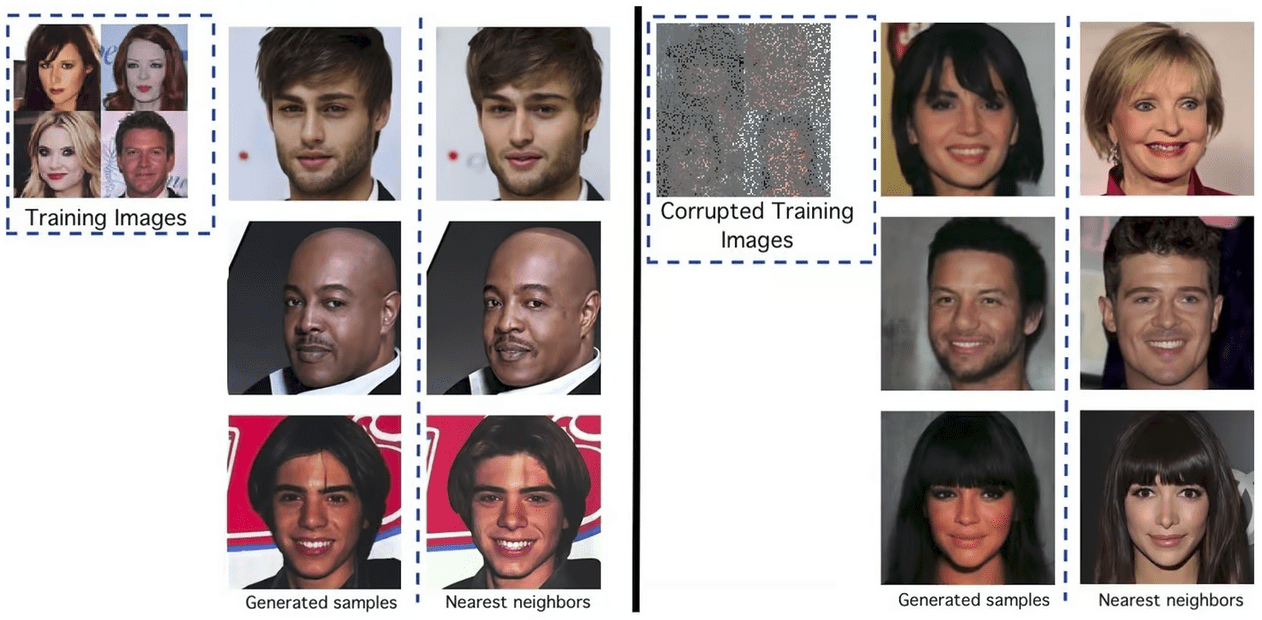
Diffusion models, advanced machine learning algorithms, progressively add noise to datasets and learn to reverse the process, generating high-quality data. However, recent studies have shown that these models can memorize examples from their training set, raising concerns regarding privacy, security, and copyright infringement. To mitigate this, the researchers employed image corruption during training.
Their experiments demonstrated the efficacy of the Ambient Diffusion framework. Initially trained on clean images from CelebA-HQ, the model replicated originals almost perfectly. However, when retrained on highly corrupted images, with up to 90% of pixels randomly masked, the resulting images were less similar to the originals, indicating a reduction in memorization and replication.
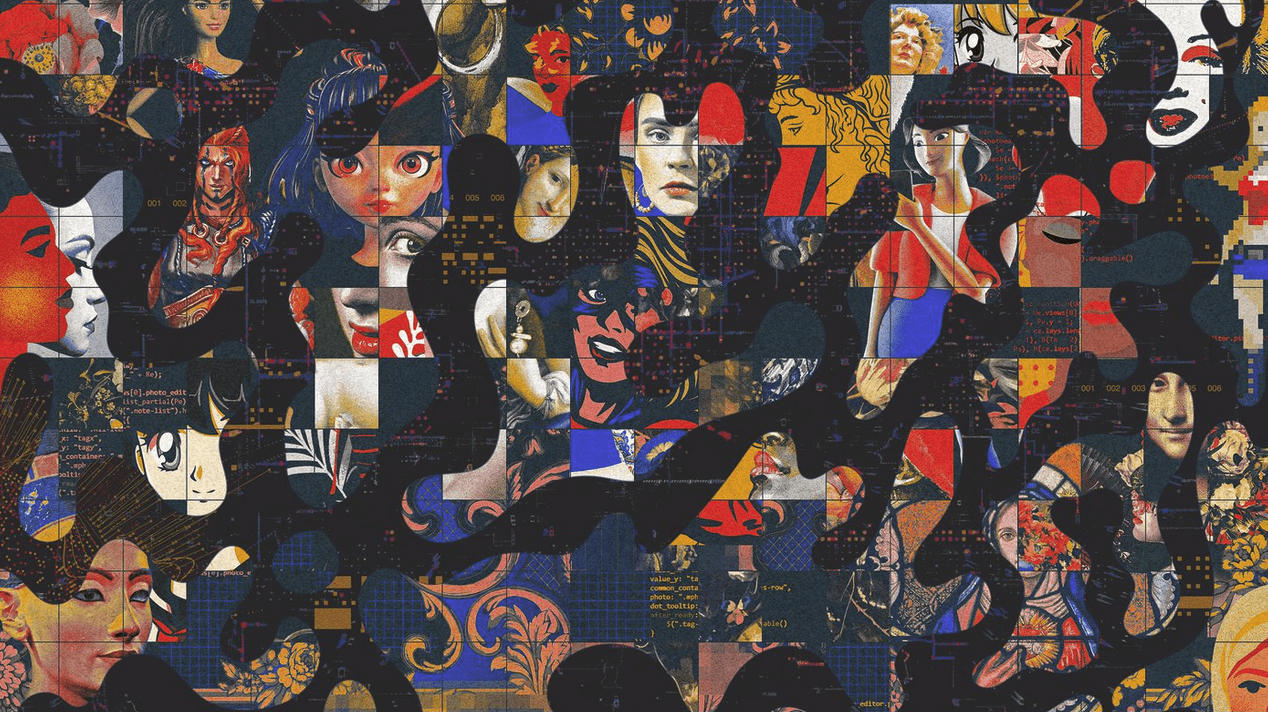
Aside from addressing copyright concerns in art, the framework holds promise for scientific and medical applications. It can be particularly valuable in fields where obtaining uncorrupted data is challenging or costly, such as black hole imaging or certain types of MRI scans.
“The framework could prove useful for scientific and medical applications, too,” said Adam Klivans, a computer science professor at UT Austin and study co-author. “That would be true for basically any research where it is expensive or impossible to have a full set of uncorrupted data, from black hole imaging to certain types of MRI scans.”
Although the results of Ambient Diffusion aren’t flawless, they provide artists with some assurance against unauthorized replication. However, this solution doesn’t prevent other AI models from memorizing and replicating original images. Legal recourse remains essential in addressing copyright infringement cases.
In the spirit of advancing research, the researchers have made their code and model open-source on GitHub, encouraging further exploration and development in this field.