In 2005, Amazon introduced the Mechanical Turk platform, a digital marketplace that offered people the opportunity to earn extra income by undertaking small tasks that required human intelligence.
These tasks ranged from data validation to simple transcriptions, which were initially considered too intricate for machines to handle. The platform took its name from an 18th-century chess-playing automaton called “The Turk,” which, despite appearing mechanical, actually concealed a person inside who controlled its movements.
As time passed and artificial intelligence (AI) chatbots grew increasingly sophisticated, a profound shift began to emerge. Researchers from the École Polytechnique Fédérale de Lausanne (EPFL) in Switzerland made a startling discovery: a significant number of Mechanical Turk workers were now harnessing the power of large language models (LLMs) to automate their labor.
“One of our key sources of human data is no longer fully ‘human!'” tweeted EFPL Ph.D. candidate Manoel Ribeiro, co-author of a yet-to-be-peer-reviewed paper on the research. “We estimate that 33-46 percent of crowd workers on MTurk used LLMs in a text production task — which may increase as ChatGPT and the like become more popular and powerful.”
In essence, humans were utilizing AI systems to streamline the execution of mundane tasks that were once believed to be beyond the capabilities of machines. Astonishingly, this trend even extended to humans employing AI models to train other AI systems, creating a peculiar hall of mirrors where AI data was utilized to educate subsequent AIs. The consequences of such a cycle were potentially far-reaching, posing risks of misinformation and chaos.
It came as no great surprise, as TechCrunch astutely observed, that AI systems had made remarkable strides in recent years. Cutting-edge tools like OpenAI’s ChatGPT and Google’s Bard had blurred the boundaries between human and machine, propelling AI technology to new heights.
In the world of Mechanical Turk, workers were remunerated on a per-task basis, often receiving meager compensation for their efforts. Consequently, when an LLM-powered tool presented an opportunity to expedite their workload, it was difficult to fault them for embracing automation.
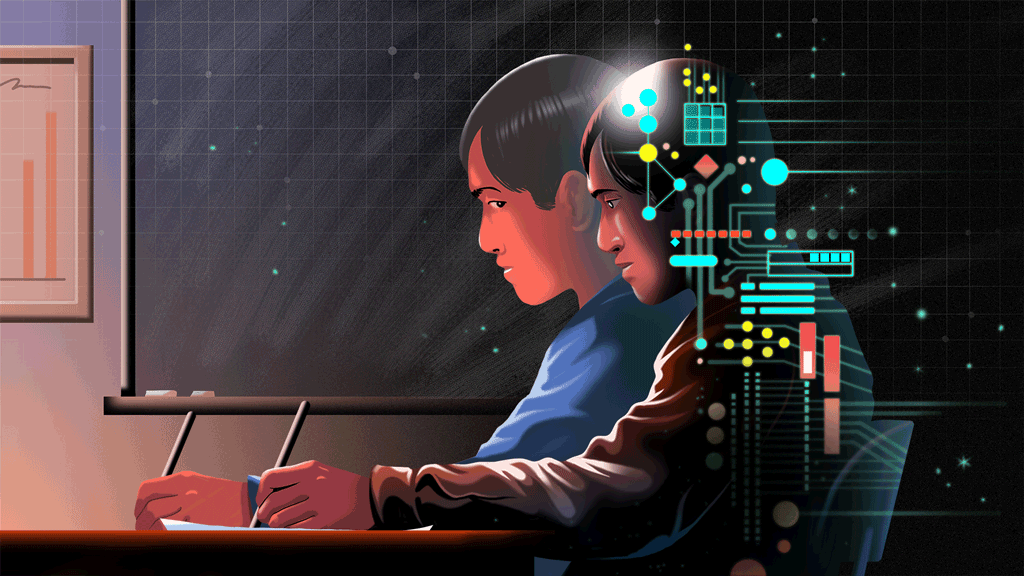
Furthermore, the lack of stringent oversight from Amazon, as highlighted by TechCrunch, likely played a role in this phenomenon.
Nevertheless, as the researchers cautioned, concerns arose as the distinction between human-generated and AI-generated data became increasingly indistinguishable. The consequences of this blurring line called into question the reliability and accuracy of the information produced, igniting apprehension about the future implications of AI-driven automation.
“LLMs are becoming more popular by the day, and multimodal models, supporting not only text, but also image and video input and output, are on the rise,” the researchers conclude in their paper. “With this, our results should be considered the ‘canary in the coal mine’ that should remind platforms, researchers, and crowd workers to find new ways to ensure that human data remain human.”