Fish fins and insect wings are marvels of natural engineering, adeptly designed for their environments to navigate through water or air. Over the years, scientists and engineers have turned to these biological models to enhance human-made machines, creating airplanes with wings and boats with fin-like rudders.
In recent decades, researchers at institutions like Caltech have delved into bioinspired engineering, exploring how the natural world’s solutions to motion and adaptation can inform advancements in mechanical design. The research, led by Mory Gharib, Ph.D., the Hans W. Liepmann Professor of Aeronautics and Medical Engineering at Caltech, investigates how robotic systems can adapt their propulsion mechanisms in response to damage, drawing inspiration from how fish and insects adjust their movement when injured. Animals like fish and insects utilize flapping motion for propulsion, and even when their fins or wings are damaged, they exhibit remarkable adaptability. Some fish can continue swimming with up to 76 percent of their fins impaired. This resilience led to the question of whether a robotic flapper could show similar adaptability.
In Gharib’s lab, researchers Meredith Hooper, an aerospace graduate student, and Isabel Scherl, a postdoctoral research associate, set out to answer this question. They tested a flapping robot in a tank of oil, which was chosen for its superior signal-to-noise ratio compared to water. After amputating a portion of the robot’s flapper, they employed machine learning to help the robot adapt its propulsion mechanism. Without help, the robot’s damaged flapper would have made it unable to move. However, by incorporating bioinspired adaptation techniques, the robot was programmed to experiment with various stroke mechanics.
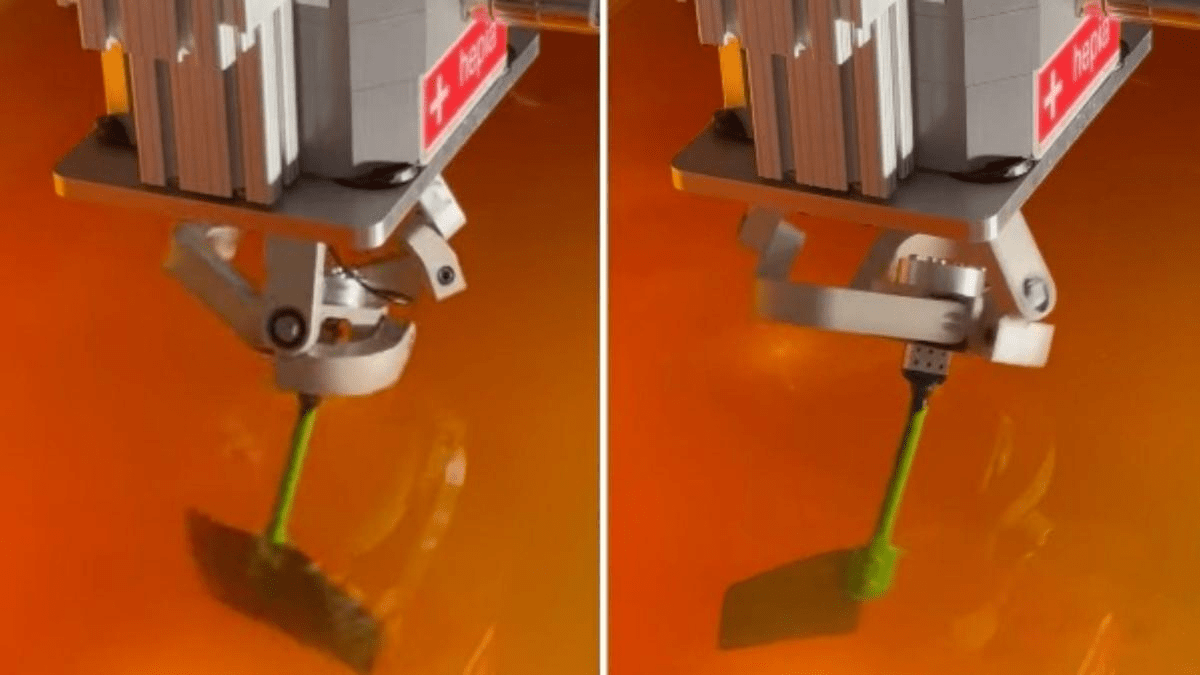
The system ran multiple trials to determine which mechanics allowed the robot to move efficiently despite the damage. Through machine learning algorithms, the robot refined its movement patterns, eventually achieving effective propulsion even with 50 percent of its flapper removed.
“The robot tries swimming in 10 different ways,” explains Hooper in the press release. “The forces while it is swimming in the oil tank are measured so that we can compare both the force production and its efficiency. The machine learning algorithm selects the top candidate trajectories based on how well they produced our desired force. The algorithm then comes up with another set of 10 trajectories inspired by the previous set.” This learning process involves repeated cycles of evaluation, modification, and creation until the top methods converge, ultimately finding the most efficient swimming motion for a given force production.
The practical applications of this research are profound. Autonomous systems, such as underwater vehicles and micro air vehicles, often face the risk of damage that could compromise their functionality. The ability to adapt to such damage could significantly enhance their utility and longevity.