In a remarkable breakthrough, researchers from the University of Texas at Austin have achieved a major advancement in brain-computer interfaces. Their latest development involves an innovative AI system, called a semantic decoder, which has the astonishing ability to translate brain activity into a continuous stream of text. What sets this system apart from other mind-reading technologies is that it operates without the need for invasive implants. This groundbreaking achievement has significant potential to assist individuals who are conscious but unable to communicate verbally due to conditions like stroke.
Unlike its counterparts, the semantic decoder developed by the UT Austin team utilizes non-invasive functional magnetic resonance imaging (fMRI) to capture brain activity and convert perceived or imagined stimuli into natural language text. Although fMRI provides exceptional image quality, it measures signals at a relatively slow pace. A neural activity impulse takes around 10 seconds to affect blood oxygen levels, while spoken English typically consists of more than two words per second. As a result, each brain image can be influenced by more than 20 words.
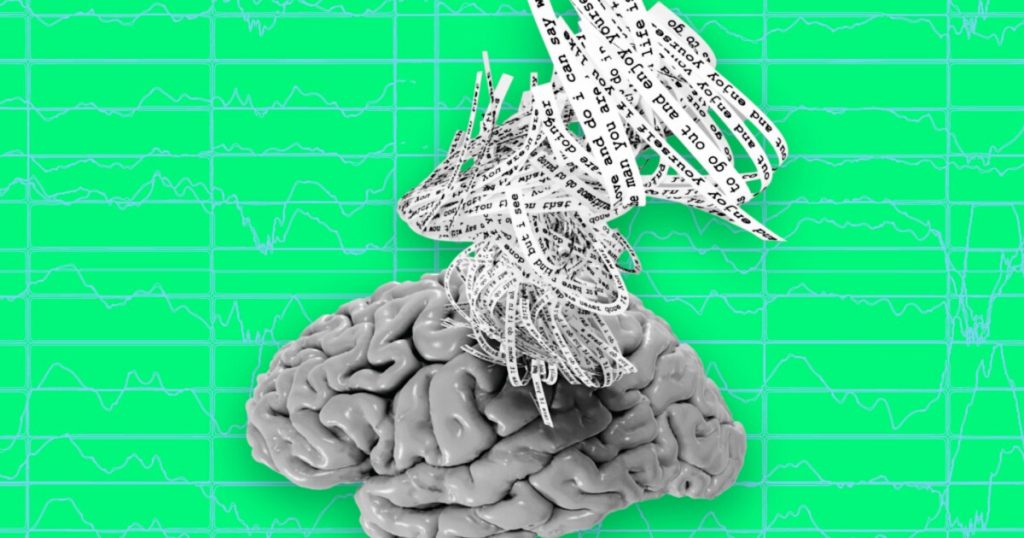
To address this challenge, the semantic decoder employs an encoding model similar to those used by existing AI systems. The researchers trained the decoder by recording brain responses of three individuals who listened to 16 hours of spoken stories. The decoder demonstrated remarkable accuracy in predicting how the participants’ brains would respond to specific word sequences. It even succeeded in capturing the essence of actively listened stories when participants ignored simultaneously played narratives.
In addition to story listening, the researchers conducted experiments where participants watched silent videos while their brain activity was monitored using fMRI. The semantic decoder impressively translated the recorded brain activity into precise descriptions of the events depicted in the videos.
However, the researchers emphasized the significance of voluntary participation and the protection of mental privacy. Results were compromised when participants exhibited resistance or focused on unrelated thoughts during training. Unintelligible outcomes were observed when testing the AI on individuals who had not undergone decoder training. The research team remains committed to ensuring ethical use of this technology, with individuals consenting and benefiting from its applications.
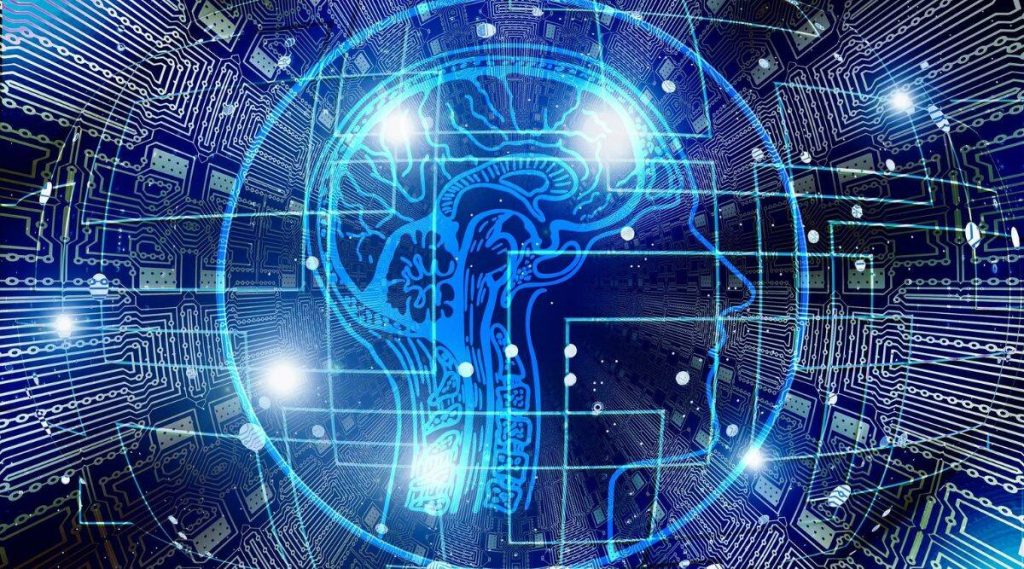
While the current version of the semantic decoder is limited to laboratory environments due to its reliance on fMRI, future adaptations could allow for portable brain imaging systems, like functional near-infrared spectroscopy (fNIRS), to be used. Such advancements would facilitate the implementation of this mind-reading AI system in real-world scenarios, potentially enhancing the quality of life for individuals with communication impairments. While there is promise for the future of this remarkable technology, it is crucial to proceed responsibly, prioritizing ethics, individual autonomy, and privacy.