Scientists at Northwestern University have developed a groundbreaking AI algorithm called MaxDiff RL that promises to outperform existing robotic systems. This algorithm encourages robots to explore their surroundings as randomly as possible, gathering a wider range of experiences for faster learning.
In tests, robots using MaxDiff RL, like NoodleBot, consistently outperformed other AI models. This new method enables robots to master new tasks flawlessly on their first attempt, unlike traditional AI that relies on slower trial-and-error learning.
The key difference lies in how robots acquire data. Traditional AI models are trained on massive amounts of human-curated data, while embodied AI systems like robots gather data independently. MaxDiff RL addresses this gap by instructing robots to move more randomly, collecting diverse data about their environment. This self-selected exploration allows robots to learn crucial skills for practical tasks.
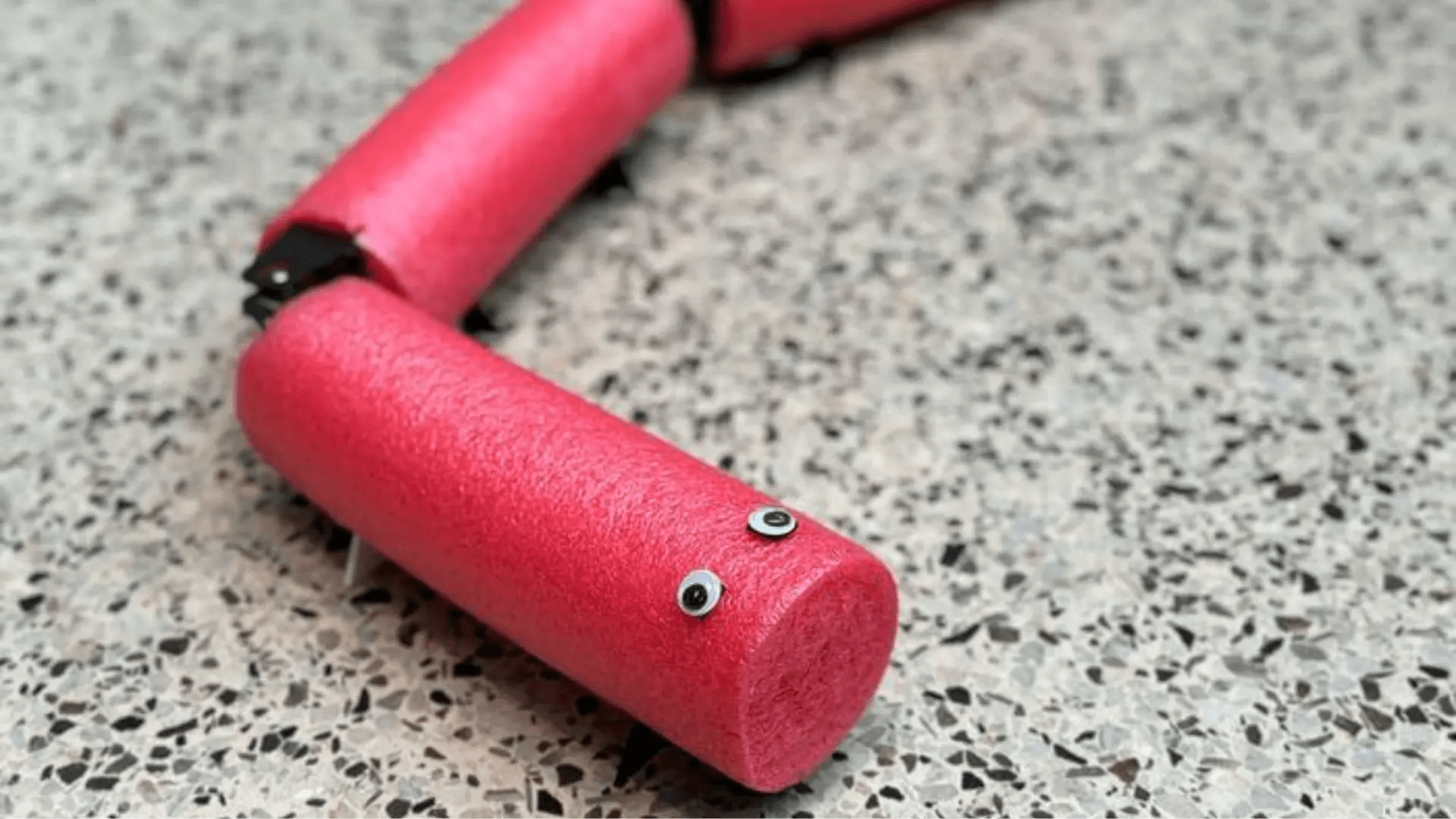
Robots using MaxDiff RL frequently achieved success on their first try, even with no prior knowledge. This makes the algorithm highly beneficial for real-world applications where time for trial-and-error is limited.
MaxDiff RL is beautiful because of how versatile it is. It is applicable to both mobile and stationary robots, such as robotic arms, and can be used for a variety of tasks. The potential of MaxDiff RL to take advantage of embodiment for faster learning becomes even more important as robotic activities and environments become more complex.
Future robotics may be considerably enhanced by this ground-breaking method, opening the door to more effective and versatile machines.