The AI revolution is here, as super-advanced machines continue to grasp the nuanced skill of being human at breakneck speed, and with the introduction of powerful AI platforms such as Open AI’s Generative Pre-training Transformer (GPT), even the boundaries between man and machine are blurring.
Michal Kosinski, a computational psychologist at Stanford University, conducted a new study in which he used several iterations of OpenAI’s GPT neural network—from GPT-1 to the most recent GPT-3.5—to perform “Theory of Mind” (ToM) tests, a series of experiments first developed in 1978 to measure the complexity of a chimp’s mind to predict the behavior of others.
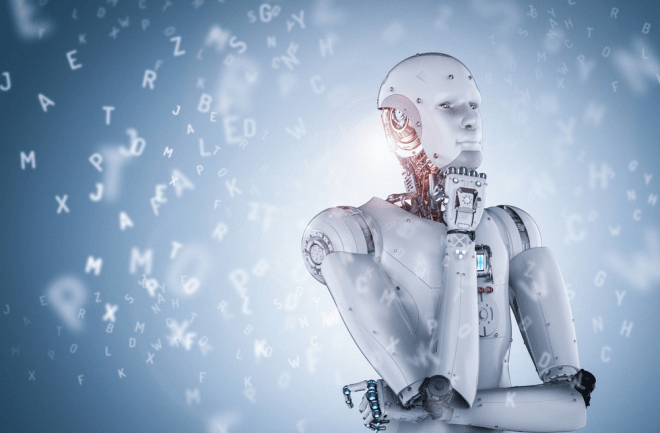
These tests entail solving ordinary, everyday circumstances from which humans may easily deduce the outcome. For example, in one scenario, a popcorn package is incorrectly labeled as “chocolate,” The AI is then asked to predict the human reaction once the bag is opened. Kosinski’s team used “sanity checks” to determine how effectively GPT networks understood the circumstance and predicted human response.
While early versions of GPT-1, launched in 2018, performed poorly on the test, the neural network demonstrated astounding improvement across iterations. It spontaneously gained the “Theory of Mind” capability of a 9-year-old human by November 2022. (the release of the latest GPT-3.5). According to Kosinski, this might be a “watershed moment” for artificial intelligence because the ability to understand and predict human behavior will make these engines far more valuable.
“The ability to impute the mental state of others would greatly improve AI’s ability to interact and communicate with humans (and each other), and enable it to develop other abilities that rely on Theory of Mind, such as empathy, moral judgment, or self-consciousness,” Kosinski writes.
The capacity to program empathy and morality could be a massive help for self-driving cars, for example, when deciding whether to put a driver in danger to save the life of a toddler crossing the street.
One unanswered point is whether these neural networks use ToM intuition or avoid it by “leveraging some unknown language patterns.” This could explain why this capacity is surfacing in language-based models that aim to understand the complex nuances of human speech.
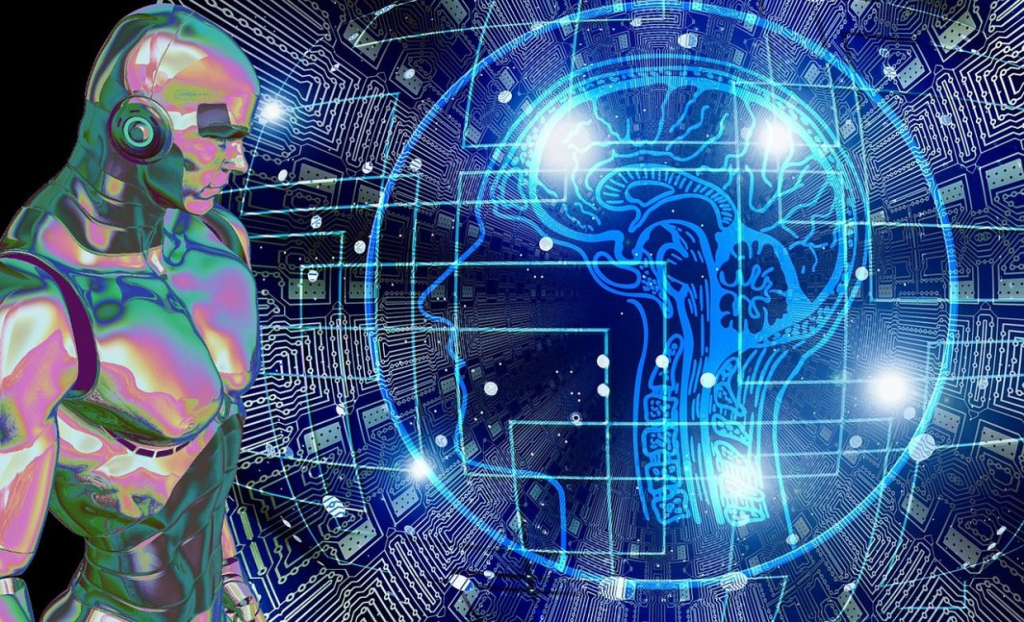
But this makes one wonder: Can people use this language trick and simply be unaware of it? According to Kosinski, by investigating the cognitive capacities of these AI systems, we are essentially studying ourselves, as the workings of the human mind remain a scientific mystery.
“Studying AI could provide insights into human cognition,” Kosinski writes. “As AI learns how to solve a broad range of problems, it may develop mechanisms akin to those employed by the human brain to solve the same problems.”
The findings of the study were made public on the pre-print portal arXiv.