A life or death situation, literally.
The Emergency Department or ED is a critical unit found in healthcare facilities that deals with all sorts of emergencies. With some acute diseases, timely admission of the patients plays a major role in higher chances of survival while negligence can result in an uneventful death and this quick identification based on priority is the job of the ED department. But to err is to human and sometimes nurses can make mistakes during diagnosis which can lead to increased mortality in patients.
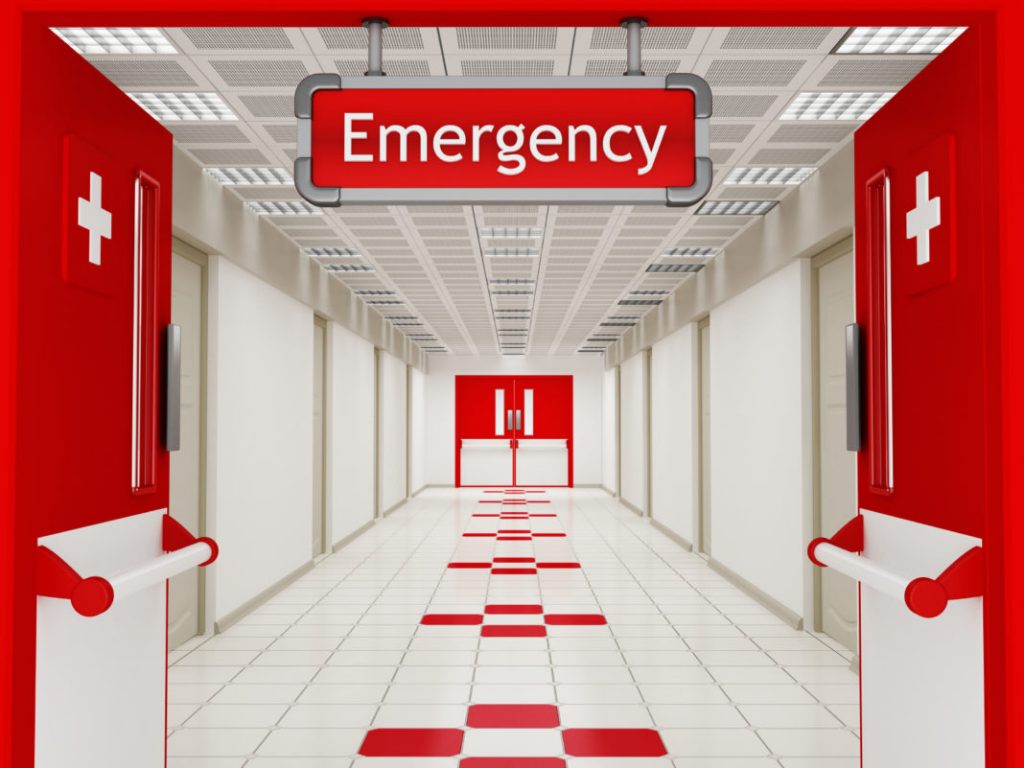
To overcome this problem, a team of researchers has published the paper titled “Using machine learning techniques to predict hospital admission at the emergency department” that proposes a machine learning tool to facilitate patient admissions in hospitals on the basis of the severity of the disease.
The research provides a valuable ML tool to promptly identify those patients that will benefit from timely hospital admissions. By using ML, the process of identifying the patients through manual diagnosis could be shifted to automatic detection of diseases and their severity which will help provide a more reliable model for admission of patients to the ED. The goal for this type of machine learning tool is to accurately identify high-risk patients and differentiate them from stable, low-risk patients that can be safely discharged from the ED.
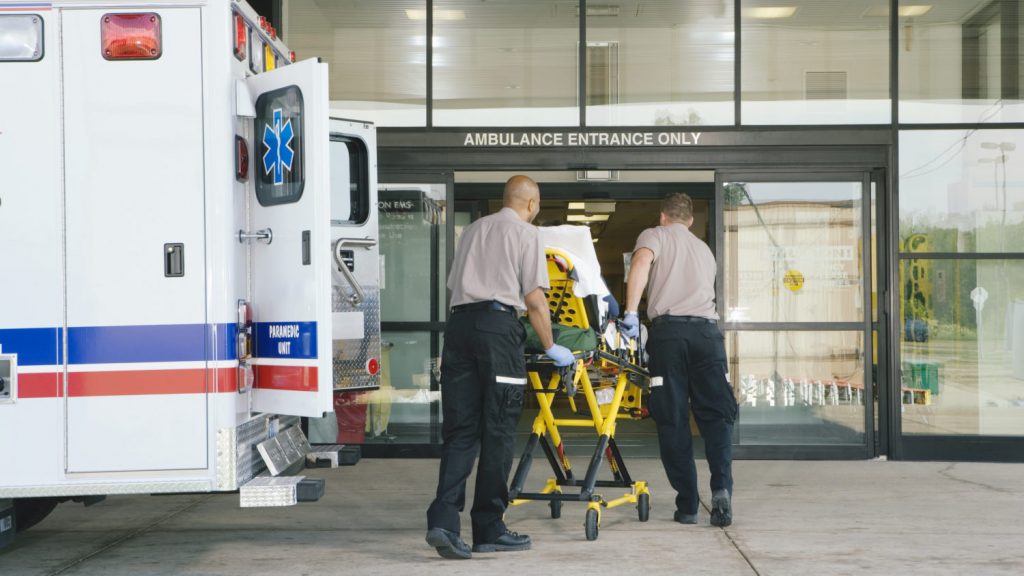
Different parameters that were investigated to predict their performance in hospital admission included serum levels of Urea, Creatinine, Lactate Dehydrogenase, Creatine Kinase, C-Reactive Protein, Complete Blood Count with differential, Activated Partial Thromboplastin Time, D-Dimer, International Normalized Ratio, age, gender, triage disposition to ED unit and ambulance utilization. The researchers evaluated 8 different ML models on the basis of accuracy which showed that this could be an effective diagnostic aid in healthcare for ED.
In the researchers’ words, “Our research confirms the prevalent current notion that the utilization of artificial intelligence may have a favorable impact on the future of emergency medicine.”