Nvidia has always been renowned among developers and computer enthusiasts, and now the global corporation is also becoming increasingly active in the car business. Consider, for example, its Drive Hyperion system, which is one of the market’s most sophisticated AV (autonomous vehicle) innovation frameworks. Nvidia has now unveiled Drive Map, a bidirectional mapping platform that is open to the whole AV industry and claims of allowing the greatest levels of flexibility. Its purpose is to increase safety on the road by assisting the AI operator in better understanding its environment. Drive Map employs mapping technologies in conjunction with an AI-powered crowdsourcing map system that collects custom maps from millions of automobiles and continually uploads fresh information to the cloud.
Nvidia’s goal is to give centimeter-level precision sample test dataset maps surveillance. By 2024, Drive Map will include 500,000 km of roads globally to offer AVs an increased recognition of their settings. The maps will continue to grow and be upgraded with millions more passenger cars over time. Street signs and altitude, islands, signage, vertical posts, and traffic signals will all be precisely replicated on the map. Through the Drive Map Stream access, any cars that satisfy the Drive Map criteria will be able to continually upgrade the map utilizing sonar, detection and ranging, and camera data.
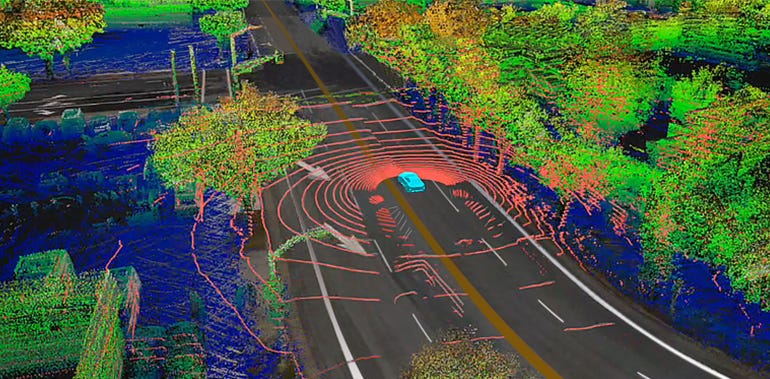
Rather than relying entirely on crowdsourcing or a formal research mapping endeavor, the DRIVE Map will be built using a hybrid of the two. This framework incorporates the perfect combination, obtaining centimeter-level accuracy while also achieving the quality and magnitude that can only be attained with billions and billions of passenger cars consistently modifying and updating the map. The acquired map data will be consolidated and placed into the NVIDIA Omniverse, where it will be utilized to refresh the map, allowing the genuine fleet to get updated over-the-air frequent updates within hours.

AV programmers can employ simulation models that combine real-world physics to build training missions that aren’t present in actual data. Finally, the virtual model might offer fleet administrators a comprehensive visual image of where their cars are driving in the world, allowing for remote monitoring as necessary. DRIVE Map, as a holistic framework, provides the AI driver with the world information required to constantly enhance automated features.