From now on, identifying the 3D shape of nearly any protein known to science will be as simple and clear.
AlphaFold, a revolutionary artificial intelligence (AI) network, was used by researchers to predict the structures of 200 million proteins from 1 million species, spanning nearly every known protein globally.
The data release will be publicly available on a database created by DeepMind, Google’s London-based AI division that built AlphaFold, and the European Molecular Biology Laboratory’s European Bioinformatics Institute (EMBL-EBI), an intergovernmental entity near Cambridge, UK.
“Essentially, you can think of it covering the entire protein universe,” DeepMind CEO Demis Hassabis said at a press briefing. “We’re at the beginning of a new era of digital biology.”
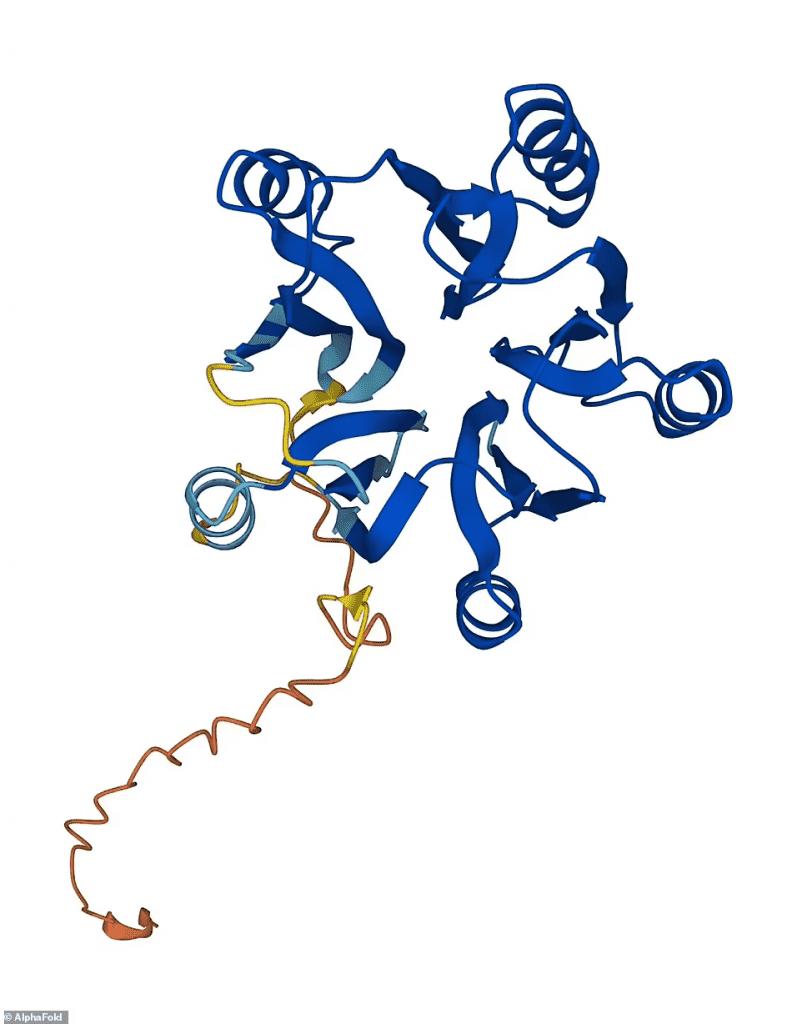
The 3D structure of a protein determines its function in cells. Therefore, precise maps are typically the first step in understanding how proteins function and most medications are developed using structural data.
The AlphaFold network was developed by DeepMind using a deep learning AI technique, and the AlphaFold database, which has 350,000 structural predictions covering nearly every protein produced by humans, mice, and 19 other widely studied animals, was made public a year ago. More than a million entries have been added to the catalog since then.
“We’re bracing ourselves for the release of this huge trove,” says Christine Orengo, a computational biologist at University College London, who has used the AlphaFold database.
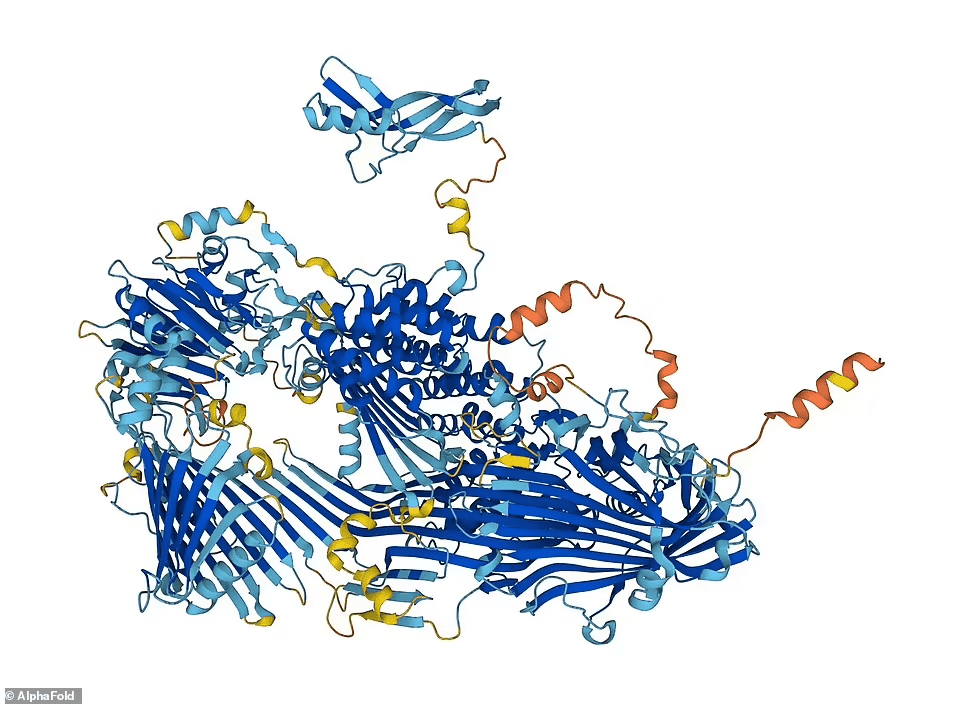
“Having all the data predicted for us is just fantastic.”
AlphaFold predicts the 3D shape or structure of proteins with high accuracy. It also offers data on the accuracy of its forecasts. Scientists have traditionally utilized time-consuming and expensive experimental approaches such as X-ray crystallography and cryo-electron microscopy to solve protein structures.
The 200 million predictions made recently are based on sequences from another database known as UNIPROT. According to Eduard Porta Pardo, a computational biologist at the Josep Carreras Leukaemia Research Institute (IJC) in Barcelona, scientists are likely to have an idea about the shape of some of these proteins because they are covered in databases of experimental structures or resemble other proteins in such repositories.
However, such entries are biased toward humans, mice, and other mammalian proteins; according to Porta; thus, the AlphaFold dump is likely to provide necessary knowledge because it draws from a broader range of animals.
“It’s going to be a fantastic resource. “But I’ll probably download it as soon as it’s available,” Porta admits.
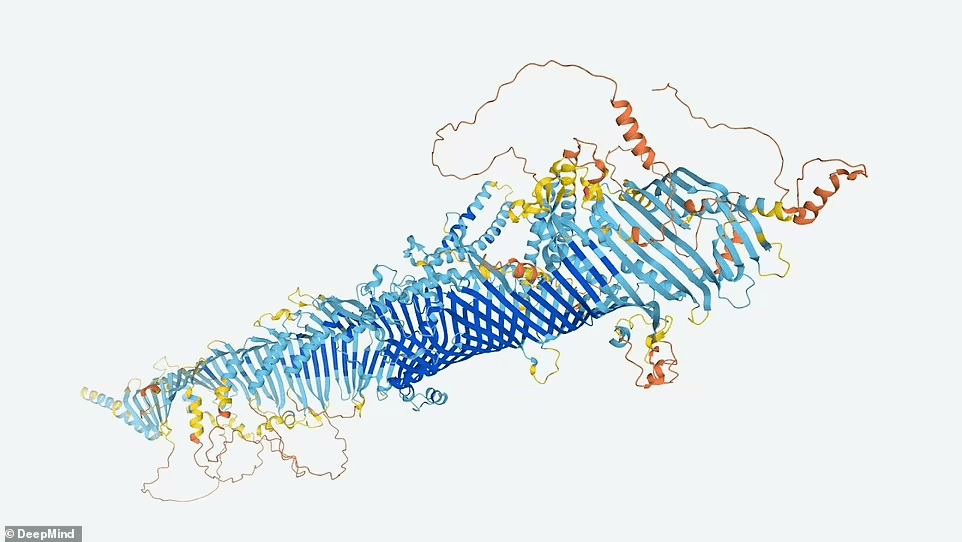
Since AlphaFold software has been available for a year, researchers can now predict any protein’s structure. However, some say that having predictions in a single database will save researchers time and money.
Jan Kosinski, a structural modeler at EMBL Hamburg in Germany, who has been administering the AlphaFold network for a year, is excited about the database expansion. His team spent three weeks estimating a pathogen’s proteome (the set of all an organism’s proteins).
“Now we only have to download all the models,” he stated.
Getting nearly every known protein in a database will also allow for new types of research. For example, Orengo’s team has used the AlphaFold database to discover new protein families and will now do it on a far larger scale.
In addition, her lab will also use the increased database to better understand the development of proteins with beneficial features, such as the ability to consume plastic, and those with concerning properties, such as those that can cause cancer. Finding distant cousins of these proteins in the database can help locate their features’ source.
Martin Steinegger, a computational biologist at Seoul National University who collaborated in developing a cloud-based version of AlphaFold, is pleased to see the database grow. However, he believes that researchers will still need to run the network.
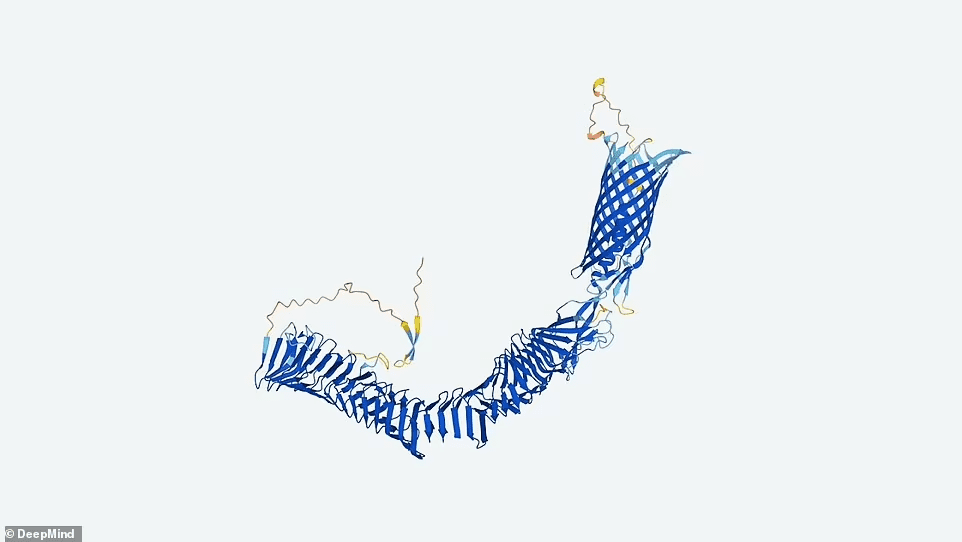
People increasingly use AlphaFold to anticipate how proteins interact, and such predictions are not in the database. Microbial proteins are also not identified by sequencing genetic material from soil, ocean water, or other metagenomic sources.
Steinegger thinks it will be costly for many to download the entire 23 terabyte contents of the new AlphaFold database to use some advanced applications. Additionally, cloud storage could be expensive.
The AlphaFold database will need to be updated when new species are discovered despite having every known protein. The predictions of AlphaFold might grow more accurate when new structural data becomes available.
According to insiders, DeepMind has promised to support the database forever and that there would be yearly updates. Experts also predict that the availability of the AlphaFold database will have a long-term effect on the biological sciences.