Streaming services and radio stations face the challenge of determining which songs will become popular hits among the tens of thousands of new songs released every day. They have tried different methods, including human reviewers and artificial intelligence, but achieving accurate predictions has been difficult, with only a 50% success rate.
However, a team of US researchers has made a groundbreaking discovery using advanced machine learning combined with brain responses to identify potential hit songs with an impressive accuracy of 97%. This innovative approach, known as neuro forecasting, analyzes brain activity data to anticipate popular music trends.
“By applying machine learning to neurophysiologic data, we could almost perfectly identify hit songs,” shared Paul Zak, a professor at Claremont Graduate University and senior author of the study, which was published in Frontiers in Artificial Intelligence.
He continued, “That the neural activity of 33 people can predict if millions of others listened to new songs is quite amazing. Nothing close to this accuracy has ever been shown before.”
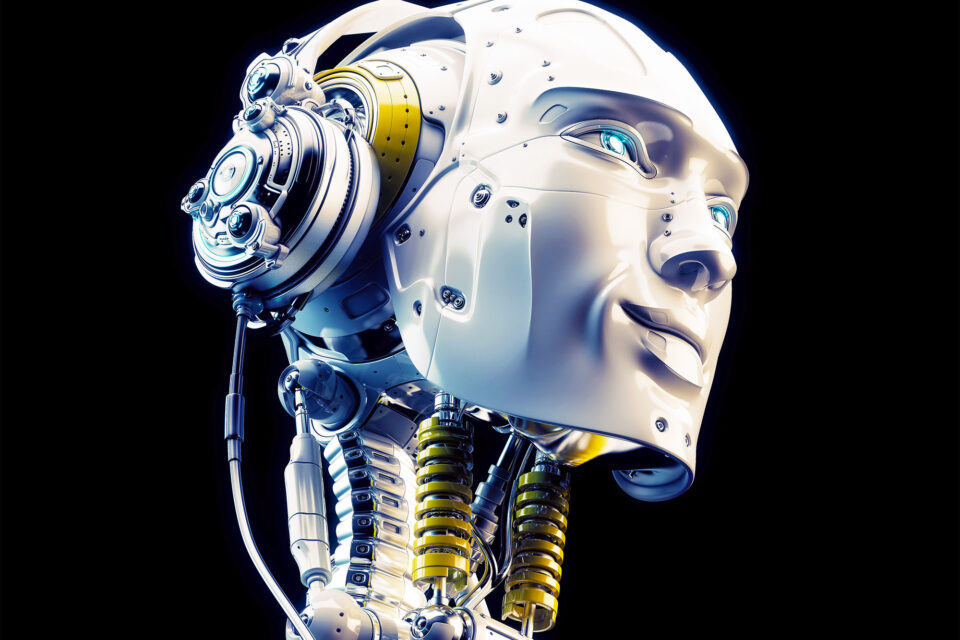
In their study, participants listened to 24 new songs while wearing sensors to measure their neurophysiological responses. The researchers collected data on their preferences and also considered demographic information. The participants’ neurophysiological responses played a crucial role in predicting a song’s success in the market and estimating the number of streams it might receive.
“The brain signals we’ve collected reflect activity of a brain network associated with mood and energy levels,” Zak explained.
After collecting the data, the researchers applied various statistical methods to assess the predictive accuracy of the neurophysiological variables. They compared different models to determine the most effective algorithms for identifying future hit songs and the results were remarkable.
While a linear statistical model had a success rate of 69% in pinpointing hit songs, applying machine learning to the same data significantly increased the success rate to 97%. Even analysing just the first minute of a song’s neural responses maintained a high success rate of 82%.
“This means that streaming services can readily identify new songs that are likely to be hits for people’s playlists more efficiently, making the streaming services’ jobs easier and delighting listeners,” Zak said.
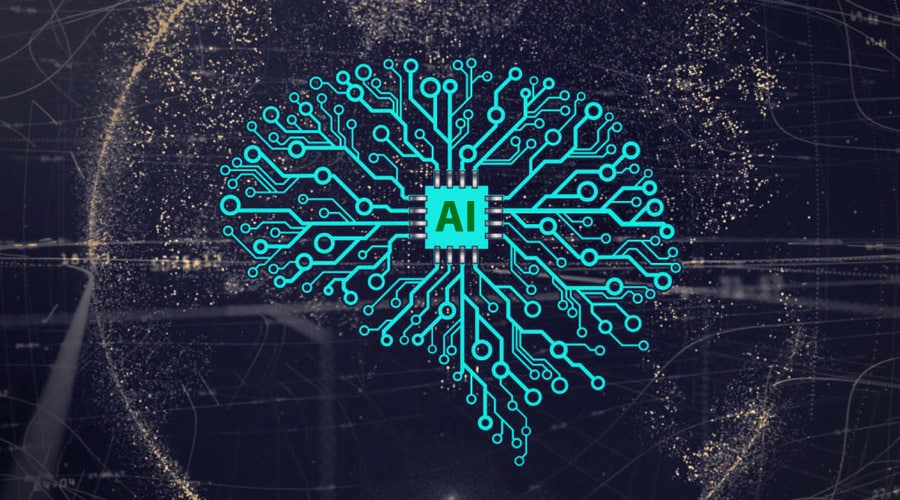
Looking ahead, the lead researcher suggested that emerging wearable neuroscience technologies could personalize entertainment options based on individual neurophysiology. This approach could reduce the overwhelming number of choices and provide listeners with quicker and more satisfying options.
However, it is important to acknowledge the study’s limitations, such as the small number of songs analyzed and the lack of diversity among the study participants in terms of ethnicity and age groups. These factors could potentially influence the results.
Despite these limitations, the researchers remain optimistic about the potential applications of this technique. In essence, this revolutionary neuroforecasting technique has the potential to transform the entertainment industry’s approach to identifying the next big hit.
“Our key contribution is the methodology. It is likely that this approach can be used to predict hits for many other kinds of entertainment too, including movies and TV shows,” Zak concluded.
Machine learning, a subset of artificial intelligence, empowers machines to learn from data and make decisions or predictions without explicit programming. It allows computers to improve their performance over time based on the data they analyze. It is of three main types:
- Supervised Learning
- Unsupervised Learning
- Reinforcement Learning